Block telecom fraud faster
Blocking telecom fraud attacks can impose a difficult decision: block faster and inflict more false positives or block slower and incur greater fraud losses. You don’t have to settle for these choices.
This paper will show you how you can block telecom revenue-sharing fraud attacks faster with fewer false positives by using the following:
- A suite of detection methods that blocks various telecom fraud attack profiles
- Self-learning fraud identification that allows precision tuning of detection supported by robust analytics
- SIP Analytics for incredibly fast telecom fraud detection
Telecom fraud detection identifies patterns of calls in fraud attacks and blocks the attack. The longer this takes, the greater the fraud losses. To minimize fraud losses, fraud analysts usually set fraud detection methods to be more sensitive.
But with greater sensitivity, most fraud systems will inadvertently identify normal calls as fraudulent. These are false positives, and blocking these calls can annoy and frustrate subscribers.
One size does not fit all
The problem is that fraudsters are clever in changing call patterns to evade detection. It’s like a game of cat-and-mouse. Looking for just one attack profile using a fixed sensitivity won’t block many telecom revenue-sharing fraud attacks. The solution: you need a suite of detection methods. Each method in this suite will:
- Look for a specific, unique calling pattern.
- Enable sensitivity settings tuned with precision to match that pattern, rather than trying to fit all patterns.
Finding their initial attack blocked, determined fraudsters will change their attack calling pattern to evade detection. But the fraudsters will only run into another detection method looking for the revised pattern. This back-and-forth will continue, with the fraudsters changing calling patterns and running into detection methods at every turn.
Eventually they give up and move to another would-be victim who isn’t so well protected. This strategy chokes off fraud attacks at every turn with minimal fraud losses and false positives. Here’s how it works.
1. Suite of detection methods to block telecom fraud
A well-balanced suite of telecom fraud detection methods will look for attack profiles that vary from very specific to broad. These methods include:
- Targeted pumping detection. Looks for high-frequency calls from one calling number to one called number. When a threshold is reached that calling pattern is quickly blocked. Many fraudsters will then turn to other expensive numbers in the same country. That’s when they run into:
- Fast traffic pumping detection. Identifies attacks from one entity to a high-cost country. When a threshold is reached, this calling pattern is quickly blocked. So, the fraudster might try to evade detection by slowing down the call frequency. That’s when they encounter:
- Slow traffic pumping detection. Catches slower attacks from one entity to a high-cost country. Foiled again. That’s when the fraudster starts jumping around to high-cost destinations across a wide variety of countries, at which point they meet:
- Theft of service detection. This method prevents telecom fraud attacks destined for any location in the world. At this point, the fraud attack has played out. Most fraudsters will give up and move on to easier prey.
Block telecom fraud attacks with a suite of detection methods
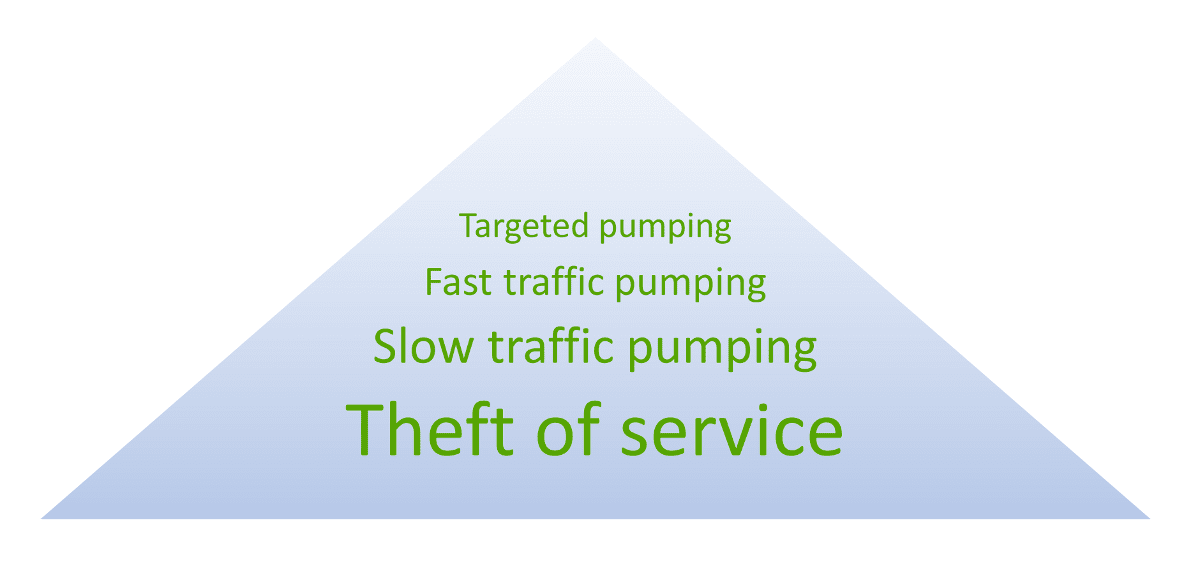
Each detection method in this suite can be precision-tuned for rapid detection and blocking with minimal false positives. The next section of this paper explains how.
2. Precision tuning of telecom fraud detection
A good telecom fraud prevention system is self-learning. This means it gathers historical call patterns for subscribers to learn what’s normal for each. If an unusual calling pattern appears, the system can block it automatically.
This is a carrier’s best protection against telecom fraud attacks. Self-learning makes the system accurate and manageable. Now fraud analysts don’t have to pour over reports and tweak settings in react mode. They can let the system manage that for them.
A good telecom fraud prevention system also allows the service provider to fine-tune the settings with simple controls in an intuitive, easy-to-use way. Effective fine-tuning requires information for decision making. The fraud prevention system should provide analytics that summarize call activity in meaningful ways to support precision blocking. These analytics should include the following:
- Trigger calibration to view summarized fraud scores, which helps visualize the line between fraud attacks and active, busy users
- Flexible reporting to find relevant information for decision making
- Charting to view data graphically, which helps identify patterns that can be difficult to notice in reports
Here is an example of a Trigger Calibration Report:
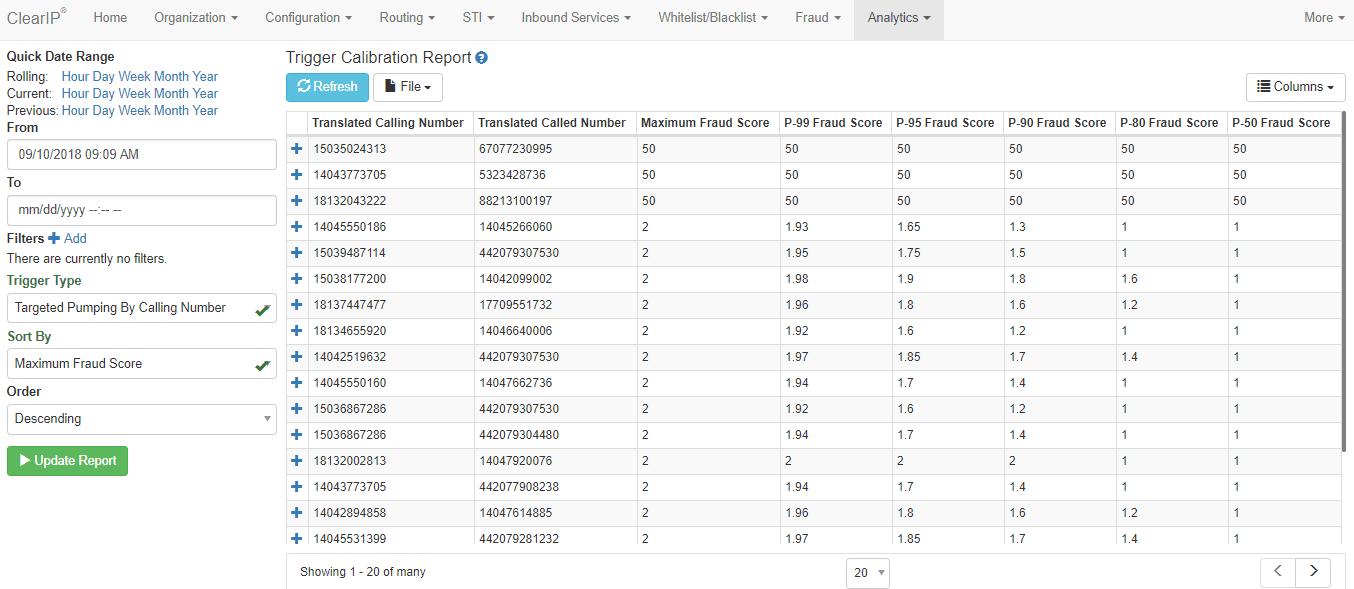
This report allows a user to review historical fraud scores for a particular telecom fraud prevention method. The report summarizes fraud scores by calling number and called number and sorts rows in descending order with the highest fraud score at the top.
In this example, notice that the first three rows had very high fraud scores. Those were fraud attempts that were blocked. The fourth row, and those that followed, were normal activity. The fraud analyst would want to make sure that, if the settings are fine-tuned, they would still properly differentiate the attacks from normal traffic. Or the analyst may decide to leave the default settings as-is. The default settings would work very well for these call patterns.
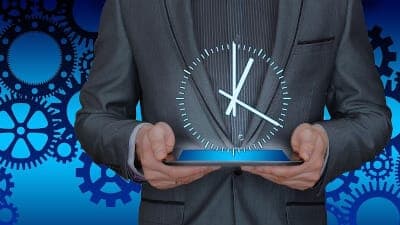
3. Faster telecom fraud detection with SIP Analytics
Deploying a suite of detection methods based upon historical average call patterns makes it very difficult for telecom fraudsters to maintain a sustained attack. But victims can still suffer fraud losses if detection time is long. Many telecom fraud prevention systems today still use Call Detail Records (CDRs) for detection. The problem with this is that a CDR is usually created after the call has ended. But fraudsters try to keep their attack calls up for as long as possible, typically 20 minutes or longer. Victims can suffer considerable losses, $15,000 or more, in the time it takes for CDR-based detection to work.
It doesn’t have to be like this. Modern telecom fraud detection studies SIP records (Session Initiation Protocol), which are created at the beginning of a call. SIP Analytics® can detect and block a telecom fraud attack in milliseconds, with negligible fraud losses.
With SIP Analytics, most customers will never know they were attacked. Their phone service will continue to work smoothly.
The following chart shows SIP Analytics in action with actual fraud attacks with a real customer:
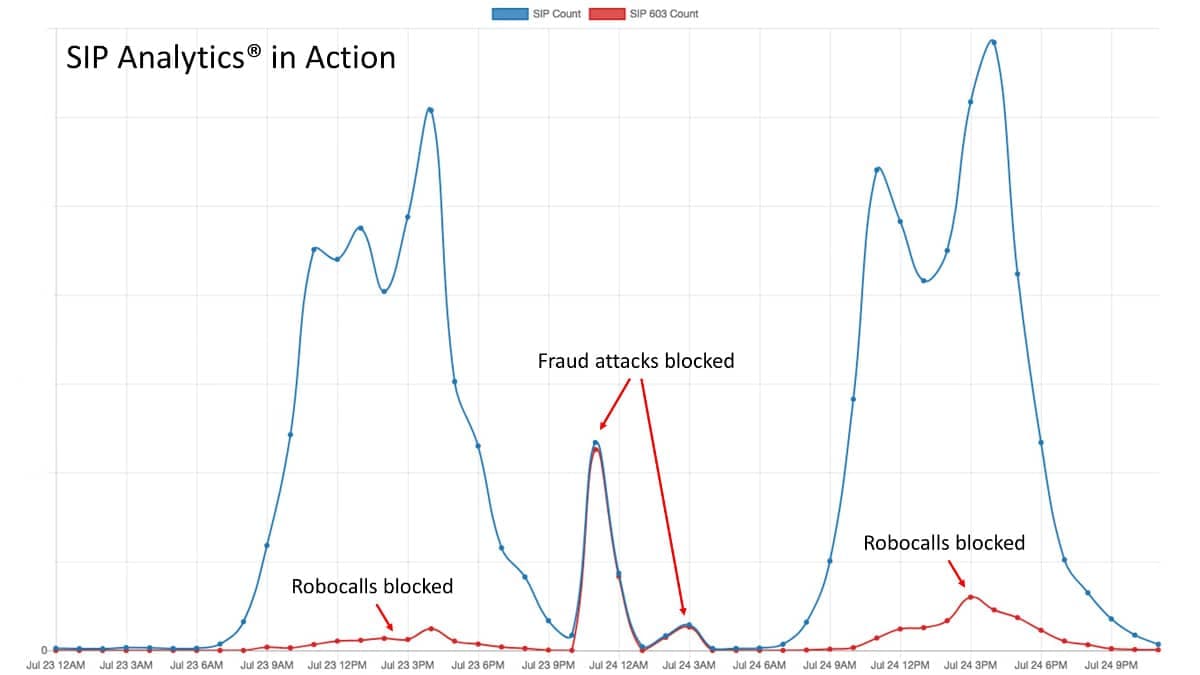
This customer experienced robocall traffic during the day and telecom fraud attacks at night. Notice how quickly SIP Analytics detected these calling patterns and shut them down. The service provider’s customers never noticed and continued to happily use the service.
CDRs are great for telecom service billing and reporting. We recommend they continue to be used for these purposes. However, CDRs should not be used for telecom fraud detection and prevention. It’s way too slow. Our customers who use SIP Analytics fraud detection would never go back to CDR-based fraud detection.
For more information, contact us today to learn how a well-balanced suite of telecom fraud detection methods can protect your network and customers from telecom fraud attacks.
More on TransNexus.com
February 15, 2023
Suggestions to curb access arbitrage
June 27, 2022
FCC proposes new rules to prevent access stimulation
June 6, 2022
Denial of service attack and ransom demand defeated
May 18, 2022
China cracks down on telecom fraud
December 6, 2021
Telecom fraud losses increasing, according to CFCA report
December 1, 2020
FCC Report and Order on one-ring scam calls
November 9, 2020
Domestic telecom toll fraud is still a problem
October 12, 2020
FCC changes rules for intercarrier compensation on toll free calls
January 23, 2020
TRACED Act calls for one-ring scam protection
November 19, 2019
Robocall and TDoS case studies
October 28, 2019
FCC denies stay request on their Access Arbitrage Order
October 28, 2019
Wangiri telecom fraud activity reported in Canada
September 26, 2019
FCC issues order to prevent access stimulation
July 16, 2019
ClearIP adds new call forwarding blacklist capabilities
July 10, 2019
July holiday week telecom fraud attack profiles
June 13, 2019
ClearIP enhancements for blacklisting of SPID and location
May 20, 2019
Anatomy of a telecom fraud attack
May 14, 2019
Study on rule changes to eliminate access arbitrage
May 8, 2019
FCC warns of Wangiri telecom fraud scams
March 8, 2019
SIP Analytics vs. CDR-based fraud management – a case study
October 22, 2018
FCC proposal to curb domestic telecom fraud
September 27, 2018
SIP Analytics® inspects each call before it begins. It’s the fastest, most precise method available to detect and prevent telecom toll fraud.
Learn more about SIP Analytics